Problems such as malfunctioning valves or internal leaks in a plant can have a significant impact on the plant and sometimes result in significant losses. To prevent such problems, Kitz developed solutions that can detect valve condition by gyro sensors.
By Isao Nishizawa, KITZ Corporation
Case study of anomaly detection by gyro sensors
We collected gyro sensors data from a customer using a valve to control slurry fluid. In this customer’s experience, the valve wasn’t able to prevent the seat breaking, or in the worst case scenario, the seat completely disappearing. Figure 1 shows an angular velocity at close to open when we first started collecting data in July. After about 4 months, we were able to detect changes in the angular velocity. Operation speed changed from 1.8 seconds in July to 2.2 seconds in December. This change was difficult to see with the naked eye.
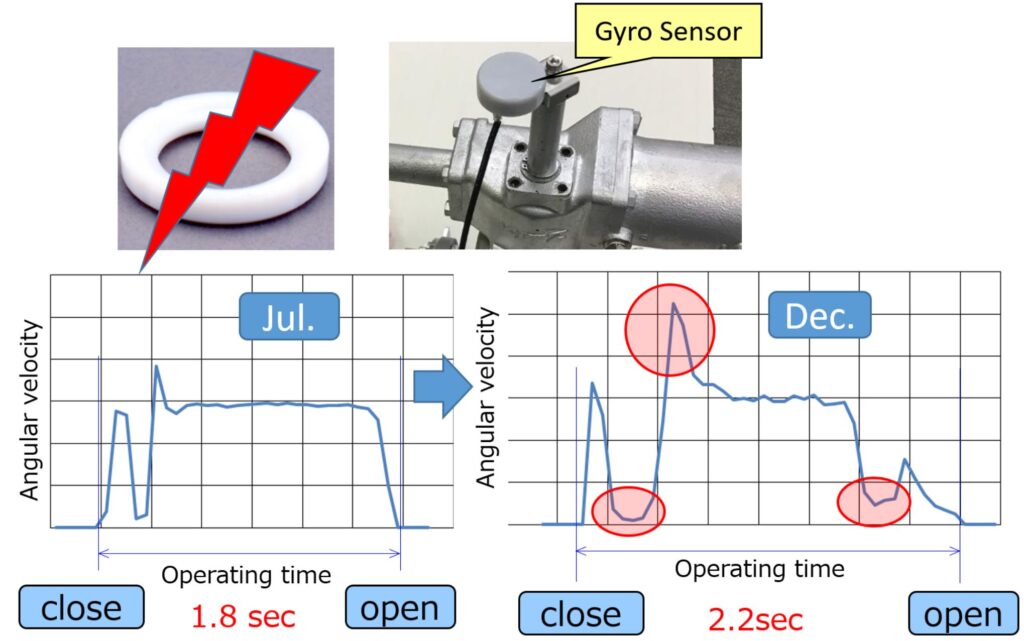
Case study of anomaly detection by machine learning
Although we found out that the angular velocities change, it is difficult to compare the angular velocities on a daily basis, and it is also difficult to evaluate them. Therefore, we visualised the difference from the angular velocity in the normal state as a score by machine learning (see Figure 2).
The vertical line shows the score value and the horizontal one shows the date. From the graph, we can see that the score changes from October to December. So, when I disassembled the valve in December and inspected it, we found that the seat was damaged. The cause of the ball seat damage is due to the slurry depositing and sticking to the back of the seat and pushing up the seat. In this situation, seat leaks were not yet occurring, so we were able to catch signs of the seat damage in advance. It was also confirmed that the score decreased after performing the maintenance of the seat replacement.
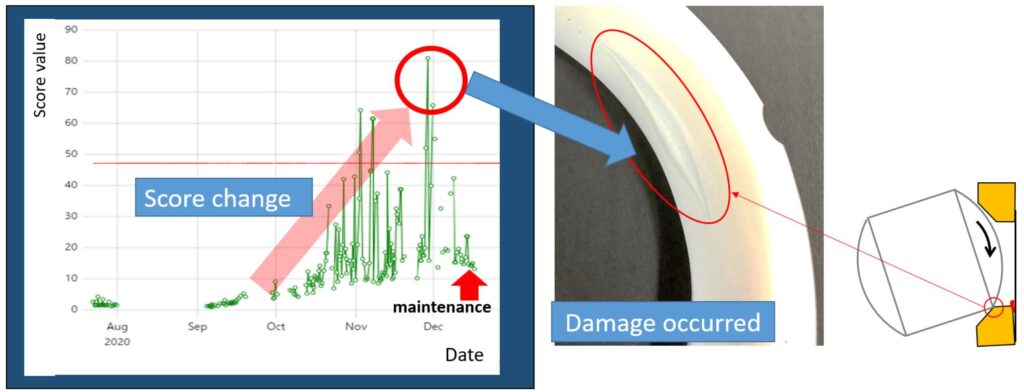
Features of the solutions
The features of our solutions are as follows (see Figure 3). Firstly, our gyro sensors were easy to install on site. Secondly, as they are powered by solar cells, there is no need to replace batteries, and they can even be used in low-light environments such as indoors. Thirdly, we used machine learning to visualise changes in the state of the valve. An overview of the system is as follow (see Figure 4).
- The sensor is easily attached to the quarter-turn valve and is powered by the solar cell.
- The data from the sensor can be transmitted wirelessly from 300m to 600m in the plant by adopting LoRaWan.
- Data is sent from the gateway to the cloud via LTE wireless communication and managed in the cloud.
- Customers can view the results of the analysis in the cloud at any time. Our service monitors collect data daily and when anomalies are detected, we will contact a customer.
Currently, in Japan, sensors have been installed in petrochemical process piping and monitoring has started
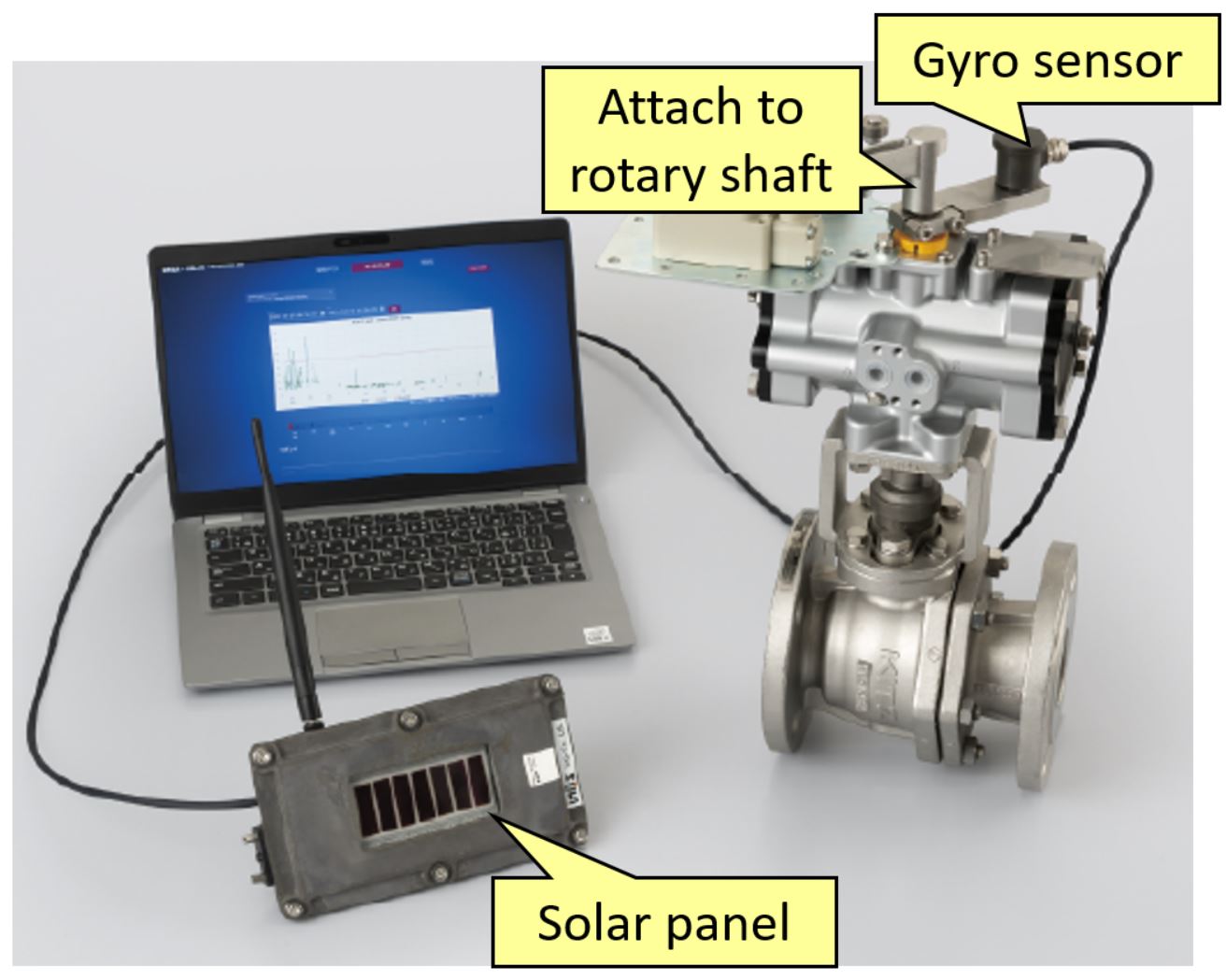
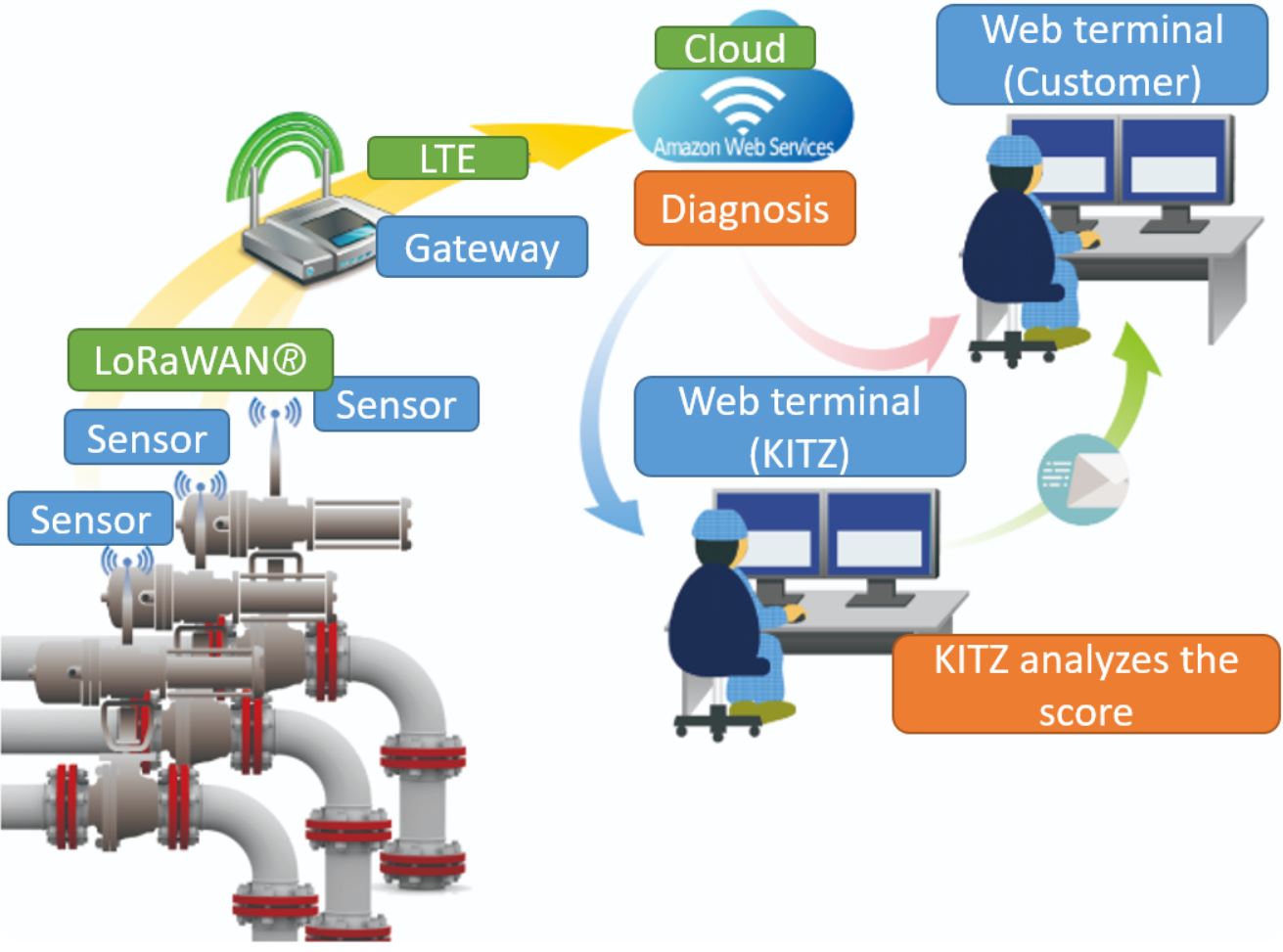